There are many pitfalls to watch out for, like the risk of using bad data (which leads to bad outcomes) or jumping on new trends without a clear plan. In this blog we want to share how to get your data ready, explore some examples from real life, and offer some helpful tips to make sure your adoption of AI is successful and delivers meaningful value.
Whether you're at the outset of your data maturity journey or looking to refine your approach, this piece provides readers with essential knowledge to avoid common mistakes, make informed decisions, and unlock the transformative power of AI in a way that aligns with your organizational goals.
Pitfall 1: Being Data "UnReady"
Research shows that 80% of companies find it hard to take advantage of AI because they struggle with organizing their data properly. Yet, preparing your data – including turning various types of data from different sources into a coordinated system - is a critical prerequisite to AI success.
Data Readiness is ultimately about having your data in a state where it can be easily used to support projects or complete analyses. Among many factors, it involves ensuring that your data is accessible, clean, and structured in a way that it can be easily and reliably integrated.
Data readiness is a foundational step for organizations looking to leverage data-driven insights and implement AI and ML projects successfully. We know that without proper data organizations risk basing decisions on flawed or inadequate information, which can lead to inefficiencies, errors, and missed opportunities.
But before working on Data Readiness initiatives, come a few important steps:
Setting Goals
You need to know what you want to do with your data. What are the organizational goals in the short term and in the long-erterm? What projects are being discussed and what is the relationship between them? This is an important on-going activity which brings together cross-functional initiatives and looks at their goals and evaluates them against your organization's current data posture, and the tools available to access the data.
These goals set the priority and direction for getting your data ready. If you're just starting to get your data in order, that's actually a good thing. While it may seem like a challenge, being early in your data management journey can actually give you a head start in many ways.
Setting Standards for Data Quality
At this point, you should focus on creating strong rules for how your data is managed. This includes organizing your data properly, which leads to better results and helps avoid problems like data breaches, legal issues, and other big risks that could hurt your business. When you work on data management, you aim to make your data as accurate, complete, consistent, and reliable as possible. High-quality data means better input for AI, which improves the results you get from it.
Protecting Data Privacy & Security
It's also vital to make rules about data safety and following the law. You'll need policies for how data is gathered, kept, processed, and shared to make sure everything you do is legal. This helps you avoid big fines and legal problems.
Setting clear goals is crucial in this process. It’s about finding a solution that aligns perfectly with your organization’s objectives and working to grow your data maturity to match the requirements of your tech choices. And don’t worry if you’re early in the data maturity journey. Though this might seem like a drawback, it offers significant advantages.
Pitfall 2: Not Managing Risk
Inadequate risk management is potentially detrimental pitfall when adopting GenAI or other AI technologies. The rapid pace at which AI and related technologies evolve can catch businesses off-guard, making it challenging to stay ahead of emerging risks and threats.
Statistics reveal a concerning trend: a substantial number of Chief Information Officers (CIOs) — 67%, to be precise — admit that their strategies for managing technology risks fall short of being effective. This shortfall is largely attributed to the dynamic nature of the tech landscape, propelled by continuous innovations and new methodologies. What's more alarming is that in 72% of cases, technology risk teams are only brought into the picture after issues have already surfaced. Furthermore, nearly half of the organizations (47%) proceed to integrate new technologies, like mobile apps and devices, into their operations without adequately assessing the risks these technologies may introduce.
To avoid falling into the trap of ineffective risk management in AI adoption, it’s crucial to adopt a proactive stance:
Proactive Risk Assessment and Management for AI
Organizations must prioritize continuous risk assessments, specifically tailored to the unique challenges posed by AI technologies. This involves a thorough evaluation of both internal processes and the external environment to identify potential threats early on. Adjusting data governance policies in response to these findings is essential. Given AI’s potential for biased decision-making and ethical concerns, it’s particularly important to scrutinize AI systems for fairness, transparency, and ethical compliance, establishing guidelines that steer AI development and deployment in a responsible direction.
Strengthening Business Continuity & AI Disaster Recovery
Developing a robust framework for business continuity and disaster recovery is indispensable, especially with AI in the mix. This strategy should account for AI-specific risks, ensuring that operations can be quickly restored with minimal impact in case of data breaches or system failures. Establishing resilient procedures for the recovery of AI systems and data integrity is crucial for minimizing downtime and maintaining trust.
The pitfall of overlooking risk management in the AI adoption process highlights the broader challenge of ensuring that AI technologies are implemented in a secure, ethical, and legally compliant manner. By focusing on comprehensive risk assessment and management, alongside effective business continuity and disaster recovery planning.
Pitfall 3: Throwing Caution to the Wind
The 3rd Pitfall is a bit of a troubling one for those of us that are early adopters of technology. There are times when innovation, without balanced by caution can create problems instead of addressing them. This is where being Data Ready (and understanding the goals of the organization) and properly assessing risk become key to not just setting up your organization for success but protecting it (and your customers) along the way.
What can we Learn From Others' Early AI Missteps?
Let's look at two examples that saw a fair bit of press to illustrate how implementing AI can go poorly without the correct preparation and constraints.
1. National Association – Chatbot Example
Diving headfirst into technology can lead to regrets, a lesson learned the hard way by a National Association in the U.S. with their chatbot. As ChatGPT sparked a chatbot craze, this association, committed to aiding its users with very specific content, announced the replacement of its human helpline staff with a chatbot. It was crafted by experts in the specified content, and was intended to be a significant and engaging resource. However, just before its implementation, a critical flaw was uncovered. The chatbot was found promoting behaviors that were significantly counterproductive, essentially fueling the harmful habits it was intended to help overcome.
This move turned out to be counterproductive for the association, not only betraying its own mission but also demoralizing its team, tarnishing its global reputation, inviting legal risks, and nearly harming the very individuals it aims to support.
2. Fortune 500 Electronics Company – ChatGPT Example
A well-known electronics company's staff, while using ChatGPT, accidentally leaked trade secrets to OpenAI. Engineers were using ChatGPT for tasks such as source code reviews, leading to three separate incidents where confidential information was inadvertently exposed. The oversight occurred because the employees didn't opt out of data sharing. This neglect led to the unintentional leakage of sensitive information, including proprietary source codes and meeting recordings. The repercussions were significant, as these corporate secrets risk being integrated into ChatGPT's future responses, accessible globally. In the hyper-competitive semiconductor industry, such data breaches can critically weaken a company's position.
As a consequence, this organization imposed a ban on ChatGPT and considered developing its own AI chatbot to prevent similar incidents. However, the damage was already done.
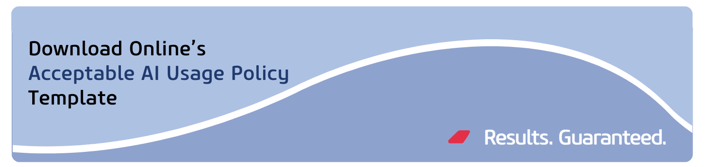
The Art of the Possible
Once you have taken the time to understand your data, properly prioritized risk management, and invested time exploring the limits of the technology, you are ready to explore The Art of the Possible. This is when the fun begins and you get to think about how to use both traditional AI/machine learning models and new, generative AI models that allow you to turn conversation into usable data, developing insights from natural language…and more.
What is the wildest, most innovative thing your organization can dream of, that solves a difficult business problem and gives you competitive advantage using data collected both inside your organization and from external sources?
Imagine that you want to grow your business, but hiring the right people quickly to staff new locations could be a barrier. You aren’t quite sure where to start and you don’t have a lot of time to generate results. Using an “Art of the Possible” approach you could look to solve this common challenge by aggregating your ‘problem’ into smaller segments, and tackling them individually to produce the best results:
- Identify a data source to leverage: Current internal employee data
- What do you want the data to do for you? Predict likelihood of success?
- Use that data to identify the desirable ‘features” you are seeking in new employees, ie: Students
- Using university and college enrollment data to identify nearby educational institutions that are within a single bus route of your upcoming new office location
- Use generative AI to develop hyper-personalized campaigns targeting each of these institutions, tailored to attract the features you have identified in this audience type
- Using generative AI to analyze applicants to find the features you are looking for and run your first campaign to test efficacy.
- Review your activity metrics and adjust accordingly to optimize your campaign.
You can then repeat this process, “connecting the dots” using other data, to identify sources of prospective customers for the new locations.
Obviously, data and organizational science work is required to identify the pieces of the puzzle needed to develop the desired insights; this is an exploration phase.
Until these needs are identified, we do not know whether we are collecting and accumulating the necessary data. As many have said, running the best data collection, cleansing and accumulation process, without having first identified those insights and required features, is like “driving a racecar to nowhere.”
In conclusion, the journey toward AI maturity is a complex yet rewarding endeavor. By focusing on building a solid foundation through comprehensive data governance, privacy, and security measures, businesses can not only mitigate risks but also harness the full power of AI to drive innovation, efficiency, and competitive advantage.
As we venture forward, the fusion of traditional and generative AI models opens up a frontier of possibilities, transforming challenges into opportunities for growth and innovation. The time to act is now, to not only prepare for the future of AI but to actively shape it, ensuring your organization not only survives but thrives in this exciting digital era.
About the Authors
Ali Farnsworth, MBA, stands as a Director, Enterprise Accounts at Online Business Systems, showcasing a robust career in technology sales that took off in a pre-IPO dot com startup in the early 2000s.
An acclaimed leader, Ali has received the prestigious Innovation in Technology Award from the National Association of Women Business Owners (NAWBO). Her contributions to the business community have also earned her the recognition as a “Women Business Leader You Should Know,” highlighting her influence and dedication to fostering growth and excellence within the tech industry.
Brian R. Spisak, PhD is an independent consultant focusing on digital transformation and workforce management in healthcare. He’s also a research associate at the National Preparedness Leadership Initiative (Harvard T.H. Chan School of Public Health, Harvard University), a faculty member at the American College of Healthcare Executives, and the author of the best-selling book, Computational Leadership: Connecting Behavioral Science and Technology to Optimize Decision-Making and Increase Profits (Wiley, 2023).
Other resources to consider:
Prompt engineering is an excellent way to fine-tune your abilities to interact with large language models, such as ChatGPT. Developing these skills is a crucial first step to take.
What is most important to take away from your experiences with prompt engineering, is to challenge the model on what it provides you with. Ask for validation of the information that it generates for you. You may be surprised at how often it adjusts its answers. Thinking of everything ChatGPT generates as a "first-draft", can help you avoid sharing misinformation and basing decisions on inaccurate data.
Effective prompt engineering enables teams and organizations to harness these newfound abilities and apply them to a broad spectrum of tasks. Familiarizing your teams with these tactics can help them become more agile, adaptive, and customer centric.
Submit a Comment